What You Should Think About When Working with Data Driven Journalism

Data journalism isn’t new at this point, but it can still be really intimidating for traditional journalists to check out. What is it? Well, data journalism, or data-driven journalism (DDJ), is “a journalistic process based on analyzing and filtering large data sets for the purpose of creating or elevating a news story.” In other words, it relies on filtering through large quantities of data that has not yet been interpreted in order to expand on or create a new topic.
Datasets are useful in all kinds of journalism, but what makes data journalism unique is that it involves an intrinsic understanding of data that may not always be intuitive. And in order to start trying out data journalism, you’ll need to have a plan.
Here’s what that might look like.
LEARN A CODING LANGUAGE
Coding languages are the backbone of automated data collection because the language determines how the data is gathered as well as presented. In order to be able to check and verify data, access to a coding language in your back pocket is really important. It will allow you to manipulate data, but also be able to cross reference it with any and all relevant points available. Python, the simplest language, is free to learn.
MAKE YOUR DATA VISUALLY ACCESSIBLE
There is a reason scientific methods of data presentation are not consumed as often as the news and it’s because, quite simply, the data itself can be too hard to interpret by just staring at it. A visualization such as a chart or a graph will be essential for you to present your findings in a way that the public can understand and accurately interpret for themselves. Using tools like the Data Visualization Catalogue can help you learn how to do this yourself or else will plot your data for you visually, which you can recreate for your piece.
KNOW THE APPROACH BEFORE YOU GET THE DATA
Datasets are tools that require a lot of inference in order to make a point. However, if the reader is inferring what to interpret from the dataset within the context of your story, you have not done your job: The inferences needed to have been made in advance, much like the data used to support your point. You can test points by inference and see if they are supported by the data. However, presenting data without a clear point of view is confusing for readers.
BE CAREFUL ABOUT WHERE YOU GET YOUR DATA AND ABOUT WHAT YOU INCLUDE
Again, there are a lot of datasets in the world. Learning how to assess for data reliability will be important, but you can preempt a lot of these issues by relying on some key sources for data. In the United States, data is usually available for free without any sort of formal process for acquiring it. But knowing exactly where that data is coming from and its objective as well as how it is being tracked, is going to tell you whether or not this source will be ultimately useful.
GIVE READERS THE TOOLS TO RECONSTRUCT YOUR RESEARCH
After you have done the work, the next step is to step back. Make sure readers are clued into all sources that were tapped for this piece and that, if necessary, the reader themselves can access all of your data sources (in addition to other sources) in order for them to be able to recreate your research. This is the strongest way to reinforce your conclusion—even if it doesn't work 100 percent of the time.
Cold hard facts are great to work with because they offer us a brief respite from the endless opinion and think pieces rampant around the internet. But even cold hard facts don’t translate into a story right away. There are many steps on the road to translating and presenting data that need to be taken for the public to be able to consume your reporting, but if you can harness those, you will have an invaluable asset on your side.
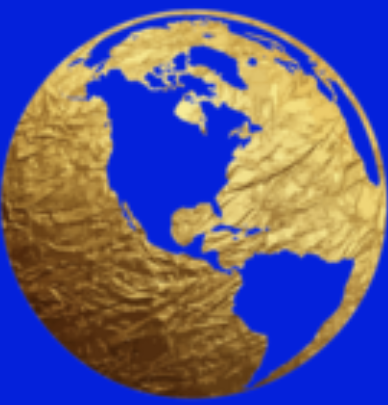