How Journalists Can Unveil Bias in AI-Generated Imagery: A Closer Look at Image Generators
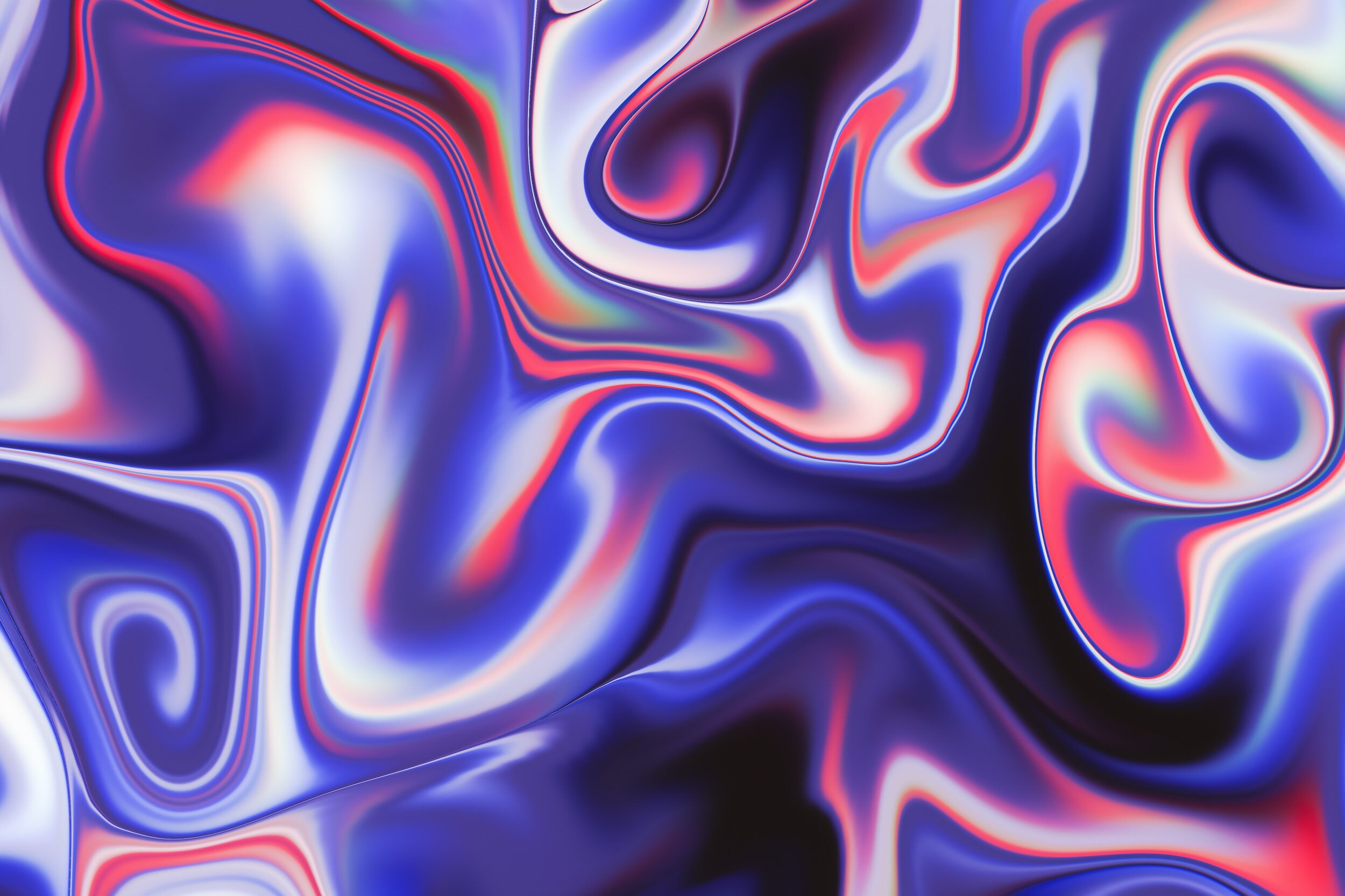
AI text-to-image generators like Midjourney and DALL-E 2 may have the ability to produce both realistic and surreal visuals, but—much like many new advances in technology—the AI is inherently biased due to the human programmer’s innate biases. AI image generators rely on machine-learning models that take text inputs and produce corresponding images. These models are trained on vast datasets containing millions of images. Many rely on a process known as diffusion, where random "noise" (aka, extraneous information added into the AI’s datasets) is added to training data, and the model learns to filter extraneous input to generate an image.
So how did bias get put into the mix? These training datasets are created by humans—who are never immune from bias—as are the algorithms underneath. Not only that, but user input is inherently biased. For instance, if users specify preferences for certain skin tones or genders in their images, the model will incorporate these preferences, and it will remember those preferences for individual users. If enough users make the same parameters, the AI will naturally generate more within those parameters even when dealing with members of the populace outside of the demographic of these initial users.
A study focusing on Midjourney’s image generation revealed that these biases were most present from both user input and the algorithm itself.
Let’s consider ageism and sexism: For any non-specified or specialized input regarding the workplace, the AI predominantly returned images of younger men and women. When the AI was asked to generate someone in a specialized role without further guidance on gender, only older men were generated. These representations reinforce harmful stereotypes, such as the idea that only older men are able to handle specialized work while women are excluded entirely, and the idea that non-specialized work is only for young people.
Furthermore, the AI exhibited serious racial bias. The images for job titles such as "journalist," "reporter," and "correspondent" featured exclusively light-skinned individuals, reflecting racial hegemony ingrained in the system. This is likely a consequence of a lack of diversity in the training data–training data input by white people, for the benefit of white people, which returns—you guessed it—white people.
Most of the generated figures lacked tattoos, piercings, unconventional hairstyles, dyed hair, or unusual clothing. In fact, overly formal clothing like buttoned shirts and neckties was prevalent, potentially reinforcing class expectations rather than accurately representing professionals' attire or the diversity of identities and approaches in the workplace. These representations of working class citizens are massively out of date, and the conservatism of the appearances themselves leads to more red flags about who has control over these algorithms.
Hilariously, the AI consistently placed the figures in urban settings with towering skyscrapers, despite over half of the world's population residing in non-urban areas. In the United States alone, 20 percent of the population lives in rural areas. Rural perceptions of elitism in urban areas could be further stoked by this bias. Additionally, the AI consistently depicts technologies from a different era, such as typewriters and printing presses, rather than current digital technologies. Whether or not this mirrors algorithmic data and input is unknown, but it is nonetheless an interesting and possibly unintentional mirror for the average age of politicians (and by default, their governing styles) in the United States.
To address bias in AI-generated imagery, users and developers must be mindful of their prompts and inputs. Consumers of AI-generated images should critically analyze the representations presented and question their representativeness of the broader population. Meanwhile, developers need to strive for more diverse and representative training data to avoid perpetuating harmful stereotypes and to make sure the AI is able to work with knowledge of present day demographics, issues, and technologies.
While AI image generators hold immense creative potential, the humans behind the AI carry the responsibility to mitigate biases that may emerge in their outputs. Humankind has a direct influence over how AI develops—and it could be the tool that ultimately breaks us, if not handled with extreme care from the outset.
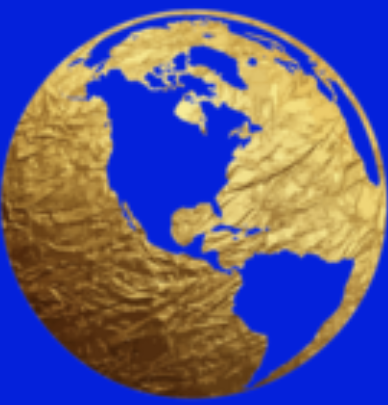