How AI and Robotics Will Transform Research Labs

A seismic shift is underway across science laboratories, where robotic automation and artificial intelligence (AI) are transforming traditional methods in chemistry, biochemistry, and materials science. Researchers at UNC-Chapel Hill propose that AI and robotic systems are not just augmenting but reshaping the very nature of experimentation, promising rapid advancements in fields as varied as health, energy, and electronics. Their paper, titled Transforming Science Labs into Automated Factories of Discovery, recently published in Science Robotics, details this visionary change.
Dr. Ron Alterovitz, the senior author and distinguished professor in computer science at UNC, explains that "the development of new molecules, materials, and chemical systems requires intensive human effort." He highlights that scientists today are tasked with painstakingly designing experiments, synthesizing materials, and analyzing results in a continuous trial-and-error cycle until they achieve desired outcomes.
This manual process, however, has its limitations, often making research slow and labor-intensive. Robotic systems offer an efficient alternative, as they can conduct experiments around the clock without fatigue, which accelerates the speed of scientific discovery. Robots bring unparalleled precision and consistency to experimental tasks and mitigate safety risks by handling hazardous materials. By offloading routine work to robots, scientists can focus on higher-level research questions, advancing crucial discoveries in medicine, energy, and sustainability at an unprecedented rate.
Dr. James Cahoon, chair of UNC’s Department of Chemistry and co-author of the study, suggests, "Robotics has the potential to turn our everyday science labs into automated 'factories' that accelerate discovery.” Yet, achieving this transformation requires creative solutions for seamless human-robot collaboration in shared lab environments. Cahoon anticipates that robotics, coupled with automation, will enhance the speed, accuracy, and reproducibility of experiments, generating vast datasets that AI can analyze to guide new lines of inquiry.
To map out the future of laboratory automation, researchers outlined five progressive levels of lab automation:
Assistive Automation (A1): Robots perform individual tasks like liquid handling, but scientists handle the majority of operations.
Partial Automation (A2): Robots complete several steps in a sequence, while humans oversee setup and monitoring.
Conditional Automation (A3): Robots manage full experimental processes but require human intervention if something unexpected arises.
High Automation (A4): Robots independently execute experiments, autonomously adjusting equipment and responding to unexpected conditions.
Full Automation (A5): Robots and AI systems function with complete autonomy, handling everything from self-maintenance to safety protocols.
These automation levels provide a framework for assessing progress in laboratory automation and establishing necessary safety standards. While lower levels of automation are already common, reaching high and full automation will require innovations in robotics that can adapt to diverse lab environments, handle complex procedures, and collaborate seamlessly with other systems and human operators.
The role of AI in the lab extends far beyond physical automation. AI algorithms can sift through vast experimental data, uncover patterns, and suggest novel research directions or compounds. Integrating AI fully into the lab workflow could revolutionize the Design-Make-Test-Analyze (DMTA) loop, where AI determines the next experiment, makes real-time adjustments, and refines the research approach. While early successes include AI’s capacity to predict chemical reactions and optimize synthesis paths, researchers caution that close monitoring is essential to avoid risks like creating hazardous materials unintentionally.
The road to fully automated labs is not without hurdles. The diverse setups of labs, from compact single-process spaces to sprawling multiroom facilities, require flexible and mobile robotic systems that can perform tasks across various stations. In addition, researchers will need training to work effectively with these advanced systems, including acquiring knowledge in data science and AI, alongside traditional scientific expertise. Preparing scientists to collaborate with engineers and data scientists will be essential to unlocking the full potential of automated laboratories.
Angelos Angelopoulos, a research assistant with Dr. Alterovitz's Computational Robotics Group and a co-author of the paper, highlights the potential impact: "By automating routine tasks and accelerating experimentation, there is great potential for creating an environment where breakthroughs occur faster, safer, and more reliably than ever before."
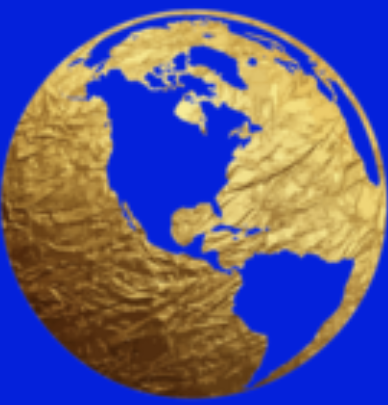